MAR 01, 2023
FAIR-Ensemble: When Fairness Naturally Emerges From Deep Ensembling
SUMMARY
Ensembling independent deep neural networks (DNNs) is a simple and effective way to improve top-line metrics and often outperforms larger single models. In this work, we explore the impact of ensembling on subgroup performances, especially when the individual models all share the same training set, architecture, and design choices e.g. optimizer and regularizers. Surprisingly, even with a homogeneous ensemble we find compelling and powerful gains in worst-k and minority group performance, i.e. fairness naturally emerges from ensembling.
We show that the minority group disproportionately benefits from ensembling, and the returns from ensembling continue for far longer than for the majority group as more models are added. While top-k performance gains plateau early after 3-4 models, we observe continual gains for bottom-k beyond 20 models in some settings. Our work establishes simple DNN ensembles can be a powerful tool for alleviating disparate impact from DNN classifiers, thus curbing algorithmic harm.
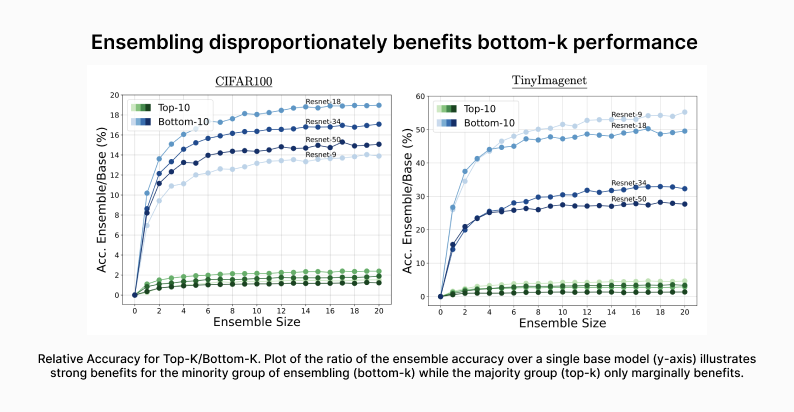
We also explore why this is the case. We find that even in homogeneous ensembles, varying the sources of stochasticity through parameter initialization, mini-batch sampling, and the data-augmentation realizations, results in differentå fairness outcomes.
KEY RESULTS
-
Simple homogeneous deep ensembles trained with the same objective, architecture and optimization settings minimize worst-case error and the gap between the bottom and top group performance as more models are added. This observation holds in both balanced and imbalanced datasets, which suggests that model ensembling can reduce the disparate impact in classification tasks.
-
Homogeneous ensembles continue to improve fairness in controlled sensitivity experiments where constructed class imbalance and data perturbation are applied. In particular, the minority group benefits more and more as the severity of the corruption increases, while the corruption has very little impact into the majority group's benefit from model ensembling.
-
We further dive into possible causes for this surprising emergence of fairness in homogeneous deep ensembles by measuring model disagreement and by ablating for the different sources of randomness e.g. weight-initialization. We obtain interesting results: certain distributions of stochasticty disproportionately benefit bottom-k performance. This suggests it is possible to improve the minority group benefits by controlling for those sources of stochasticity between the individual models.
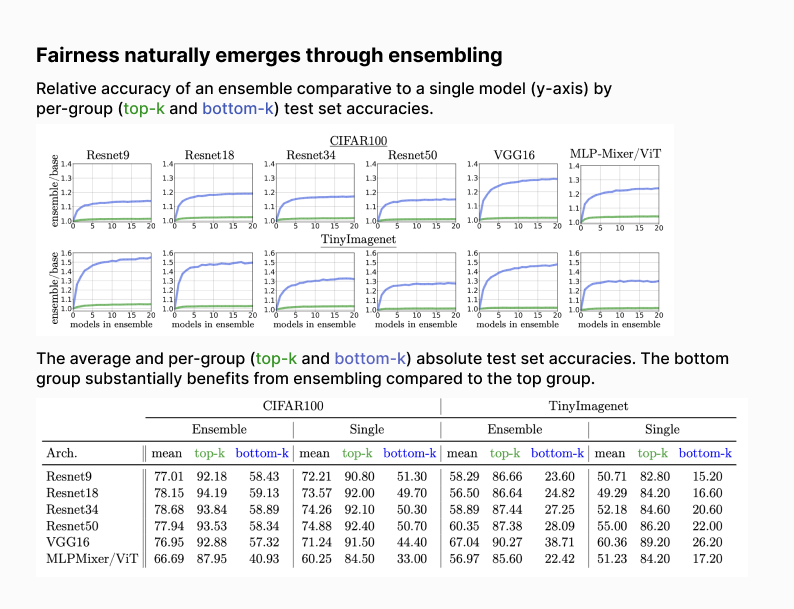